Artificial intelligence-powered models, systems and technology have the potential to significantly improve the management of occupational safety and health (OSH) risks, but it is vital that OSH practitioners understand the limitations and dangers of using AI to protect people at work.
Features
Why quality data is essential when using AI for occupational safety and health management
It is always fascinating to think that a typical adult human body consists of about 30 trillion cells and that they developed from a single cell. How these 30 trillion cells (and different organs made from them) behave and perform their functions is written in genetic codes made of proteins.
In simple terms, our genomic data, in combination with our brain and its neural network consisting of approximately 80 billion neurones, controls the functions of and interactions between different organs of this intelligent computing machine. Simply speaking, plenty of precisely sequenced proteins, electrochemical reactions, signalling and switching systems manipulate the data and its closely associated information to bring and maintain order.
The competence of this machine improves over time with the aid of more data and information and the ability to analyse them and undertake deductive reasoning. If something goes wrong in the data sequencing and communication, a person can end up with problems – for example, work-related lung cancer and allergic contact dermatitis.
So, isn’t it captivating to think that much of the ideas, intelligence and information that led to the development of modern AI models and their machine learning artificial neural networks (see Figure 1) were learnt from understanding the science involved in developmental biology? Much more will be learnt, and the new information will be used in the development of future AI models.
Figure 1: This figure shows an illustration of an artificial neural network involved in deep machine learning (DL). The interconnecting lines depict the connections between nodes and the complexities involved in signal communication.
Data and information
Like the human body, for an AI model to function correctly, among other things, it depends on quality data, the ‘fuel’ of AI models.
The terms ‘data’ and ‘information’ are often used interchangeably, giving rise to confusion. In this article, data means raw facts and figures that do not make adequate sense or do not have much meaning for decision-making. (For example, data arising from the use of respiratory protective equipment (RPE), indicated as just a simple ‘yes’ or ‘no’.) In the case of information, the data is organised, structured and processed in order for it to make sense and to gain insights and make decisions. (For example, a fit-tested half-mask respirator with high efficiency dual filters was used for protection against silica dust and in association with on-tool dust extraction, and data was recorded about its use).
In turn this led to the creation of reasonable information/‘data’, potentially usable for training an algorithm to create an AI model for pattern recognition in workplaces. Although one can argue that the information as given lacks further detail (for example, was the RPE worn correctly? Was the user trained on correct use? Was the RPE stored correctly? and so on), the information/’data’ as available is much more meaningful and useable than just ‘RPE used – yes or no’.
Although the philosophical thinking about AI may have been born before the Christian Era, modern AI-based systems are only several decades old and are developing at breakneck speeds. Photograph: iStock
Biased data
Occupational safety and health (OSH) practitioners should be mindful of the real potential for data and AI-systems-led biases when undertaking risk management decisions based on AI models’ outputs.
Some models, for example, may contain demographic differentials because the models did not have representative data for making reliable decisions about a workforce which is ethnically diverse. For example, if a biased AI model is deployed at construction sites for assessing personal protective equipment (PPE) selection, PPE use compliance, and tracking PPE dispensing patterns from PPE dispensing machines, there could be problems with the suitability of the PPE issued and the accuracy of any assessments made about user acceptance and correct use of the equipment. The meaning of bias includes the actions of supporting, opposing or thinking in unfair ways.
Figure 2 shows examples of commonly encountered biases that can impact the correct performance of AI models.
Figure 2: Examples of commonly encountered AI systems-associated biases. A further discussion is in the source material.
Therefore, if an OSH practitioner is using an AI system/model, they should seek assurance from the AI system/model vendor that the AI system/model in use has been trained and validated using good quality data that is relevant to the specific OSH application the practitioner is seeking to assess and manage. It also makes sense for OSH practitioners to ask several searching questions (see the topics in Figure 2) to help minimise bias in AI models used in OSH.
In addition, AI models should be regularly calibrated (using quality data) to ensure the model remains reliable for the practitioner’s specific operational environment in the workplace – in terms of its transparency, accountability, safety, explainability and fairness to those subjected to the model’s intelligent decision-making.
In this context, it is worth considering the practical value of accreditation approaches and the Health and Safety Executive’s (HSE’s) recommendation about accreditation to ISO 45001 – (“…. in some respects, it [ISO 45001] goes beyond what the law requires, so consider carefully whether to adopt it.”).
When preparing to seek accreditation to ISO 42001 (an international standard governing AI management systems), OSH practitioners may take a similar view as HSE. First, most OSH practitioners are not trained to be competent experts in the development and the technical management of AI systems, so they should seek help from persons who are competent in AI systems development and management.
Secondly, accreditation does not ensure that an AI model’s development and its use involved drawing on quality data. Instead, accreditation helps to ensure that the way an AI model is used at a specific workplace is done the same way day in and day out.
Here is an example: an operator was crushed to death by an AI-powered robot that failed to differentiate the human operator from the boxes of food it was designed to handle. One of the reasons for the accident would be a close association with the judgments arrived at from the input data, its decoding, reading and interpretation by the AI model for its probability-based pattern recognition.
An OSH practitioner must never take it for granted that an AI vendor’s sales and marketing technique, known as ‘AIDA’ – creating attention to their model, creating interest in their model, creating a desire in customers to buy their model, and achieving the sale of their model – is the guarantee of data quality.
Dr Bob Rajan-Sithamparanadarajah: "OSH practitioners should be mindful of the real potential for data and AI-systems-led biases when undertaking risk management decisions."
About AI
As we explore more examples to illustrate the importance of quality data, I will provide a brief background to certain AI-related matters. Although the philosophical thinking about AI may have been born before the Christian Era, modern AI-based systems are only several decades old and are developing at breakneck speeds. During the past 50 years or so, several definitions and explanations have been proposed for the term AI. Briefly speaking, (as already alluded to), ‘AI model’ means it thinks and acts almost like a human. Reflect on this explanation when reading the paragraphs in the introduction to this article.
In a nutshell, an AI system, which includes hardware, software and models, as well as data, is the outcome of an integration of various aspects –such as cognitive science, computer science, physics, mathematical science, data science, engineering principles and design technology.
The block diagram in Figure 3 provides an overview of a typical AI model development and deployment, with OSH context in mind.
Figure 3: A simplified block diagram shows how an AI model is developed for deployment. The part of the diagram above the dotted line describes the model development, and the diagram below the dotted line describes the model deployment.
In an AI system, the model determines the capabilities, functionalities and accuracy of the outputs, and the overall process involved bears a resemblance to the ‘Plan, Do, Check, Act’ cycle in management.
In essence, based on its training, an AI model acts as a probability-based pattern recogniser to provide answers to input prompts. For example, if an appropriately chosen algorithm is trained to recognise different types of tower cranes, their installation and operation, the resulting AI model should be capable of recognising the relevant features of a tower crane in a specific work situation where the OSH practitioner is managing the operation’s OSH risks.
Types of AI technology
Like AI definition, AI technology is classified in several ways. Figures 4 and 5 summarise AI’s taxonomy based on capabilities and functionalities, respectively.
Figure 4: Types of AI based on capability (i.e. the ability to do things).
With reference to Figure 4: A narrow or weak AI is designed to perform a specific task or closely related specific tasks. It means a weak AI system can only perform the task according to its design, programming and training. Almost all types of AI in use today fall into this capability category. Examples of weak AI systems include virtual assistants available via a mobile phone, spot-welding robots and autonomous vehicles such as self-driving forklift trucks and cars. These types of vehicle use trained AI models to interpret the input data (for example, from cameras and
GPS signals) to make real-time control decisions.
However, there was a real-life incident where an autonomous car’s sensing system failed to recognise a pedestrian crossing a road, resulting in a fatal accident. The circumstances point to the fact that the AI model that resulted from the training data (as well as the input data), was not of sufficient quality to enable accurate and precise probability-based pattern recognition.
A strong AI, or artificial general intelligence (AGI), aims to replicate human intelligence to perform intellectual tasks like a human. It means a strong AI model would be capable of using its learning, skills developed, experiences and cognitive flexibilities to perform new tasks in different contexts without human intervention. Unlike humans, AGIs don’t experience fatigue or have emotional and biological needs. These kinds of AI models may surface on the market soon. A possible example would be an AGI model sets up a tower crane at a construction site, tests that the crane has been erected correctly, and then hands it over to a weak AI model to operate the crane to perform lifting tasks. However, this type of scenario is many years away and many practical and operational challenges have to be addressed when developing AI models (for example, the topography of the site where the crane is to be installed).
A super AI, if realised, would surpass human cognitive abilities to think, reason, learn and make judgments independently. A super AI would have its own needs, emotions, temperaments, abilities, etc.
A frightening thought!
Figure 5: This figure illustrates the types of AI systems based on functionality to do practical tasks using digitally enabled technologies (DETs) and AI model(s).
With reference to Figure 5 and functionality-based taxonomy:
A reactive AI is a kind of rule-based expert system. It performs specific and specialised tasks based on what it has learnt from a large amount of data and statistical analysis. Reactive AI models do not have memory of past events (i.e., inputs received from past users), and they cannot recall past experiences to inform and influence current decisions.
This type of AI has existed for many decades, like the ‘Deep Blue’ chess-playing computer model that beat Garry Kasparov in 1996. Many OSH practitioners will be familiar with the RPE selector tool developed by HSE and Healthy Working Lives Scotland. In this case, the quality of the data, rules and the model’s accuracy and precision were assured by specialist experts in RPE selection and use. Software programmers created the rules-based model. The performance of the model was validated using hundreds of case studies.
A limited memory AI can recall past events and use present data to make decisions. It learns from stored and present data to improve performance over time. A common example is an autonomous motor vehicle. In this case, the vehicle’s sensors collect data around them and make decisions such as limiting the speed of travel based on national speed limit information gathered from GPS data. In a similar way, some AI-powered drones instantaneously adjust their flying operation to suit prevailing environmental conditions.
Human-led meaningful control
To minimise AI model ‘hallucination’, models should be trained and validated using a large pool of good quality data. Consider an example that relates to professional competence. A lawyer got into legal difficulties as a result of using an AI model designed for legal research. The model produced legal cases that did not exist and was spotted by a keen-eyed judge.
So, consider the risk of this happening when, for example, writing an essay for a professional membership examination or CPD submission. Recently, a colleague got caught using ‘AI-hallucinated’ data in an essay.
Unethical persons could use AI models and data for abusive purposes – for example, AI-generated child sex abuse images. Therefore, the use and application of AI models for OSH management purposes in the workplace should operate within a meaningful human-led control (i.e. responsible human-in-the-loop), instead of letting the models and bad actors play their games without adequate and suitable oversight. Just imagine the scenario of someone at work manipulating the data to harm a colleague.
If applications of AI models at work are not diligently managed, there is a significant potential for work-related stress arising among workers. For example, a company was recently fined for ‘excessive’ surveillance of its workers, including measures the data watchdog found to be illegal.
In this case, the employer’s AI system tracked employee activities so precisely that it led to workers having to potentially justify each rest break.
One way of managing this issue is to ensure that the contributory components (i.e. AI models and the associated systems, work tasks/processes, work environments and workers) always function safely and securely. Furthermore, actions should be taken to ensure that the ‘perceived safety’ of workers (comfort, predictability of the behaviours of AI systems, transparency of operation during use, sense of control among workers, their trust in the system, and their experience and familiarity to operate the system) forms part of overall risk management.
Open-access general-purpose large language models
OSH practitioners should remember that when using general-purpose, open-access large language models (LLMs), like chatbots, they will often come across limitations.
For example, if someone asks, “What respirator should I use against ammonia fume?” or “What type of local exhaust ventilation (LEV) system should I use for controlling exposure to ammonia fume?”, at present, the answers they get may not be as good as the ones they will receive from HSE’s RPE or LEV guidance booklets. Furthermore, an OSH practitioner would get better support from the reactive AI-RPE selector tool developed by HSE and Healthy Working Lives and HSE’s web-based COSHH-Essentials direct advice sheets.
In other words, AI models are not a panacea but are tools in an OSH practitioner’s safety management toolbox. They should be used in conjunction with professional competence and within the bounds of applicable legislative principles.
Conclusions
Increasingly, OSH professionals are using digitally enabled technologies (DETs) and the associated AI systems to perform a variety of OSH risk management tasks. The Alan Turing Institute, in association with the UK Government, has published guidance to ensure that non-technical employees and decision-makers understand the opportunities, limitations and ethics of using AI in business settings. It is a good idea to read this guidance.
At the same time, it must be noted that if AI systems are developed, implemented and used without necessary governance processes for safe and ethical operation, they can harm people at work, as well as the reputation and wellbeing of organisations. As discussed in this article, one of the key quality determinants of any AI system is the quality of data, which is the ‘fuel’ of AI models.
Dr Bob Rajan-Sithamparanadarajah OBE JP PhD is Vice president of Safety Groups UK (SGUK)
He is a former principal inspector and principal strategist for the UK’s Health and Safety Executive (HSE). He was one of the key developers of the award-winning LOcHER (Learning Occupational Health by Experiencing Risks), a global programme designed to allow young apprentices and students to learn and apply occupational health risk control practices.
Inspiration for this article came from his book on AI in Occupational Safety and Health
FEATURES
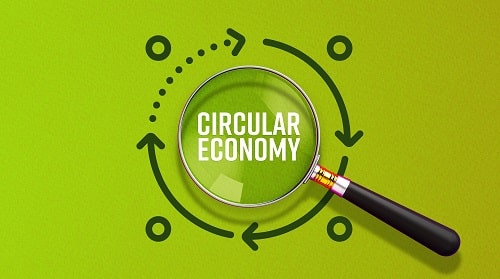
How to build circular economy business models
By Chloe Miller, CC Consulting on 07 April 2025
Widespread adoption of a circular economy model by business would ensure greater environmental and economic value is extracted and retained from raw materials and products, while simultaneously reducing carbon emissions, protecting the environment and boosting business efficiency and reputation.
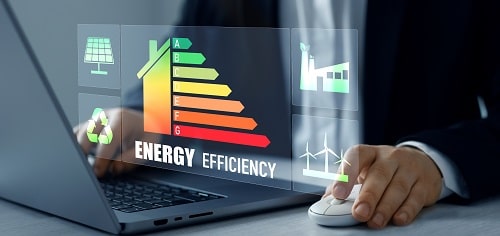
What does the first year on an accelerated net zero path have in store for UK businesses?
By Team Energy on 07 April 2025
The UK is halfway to net zero by 2050 and on a new, sped-up net zero pathway. In light of this, Graham Paul, sales, marketing & client services director at TEAM Energy, speaks to TEAM Energy’s efficiency and carbon reduction experts about the future of energy efficiency and net zero in the UK.
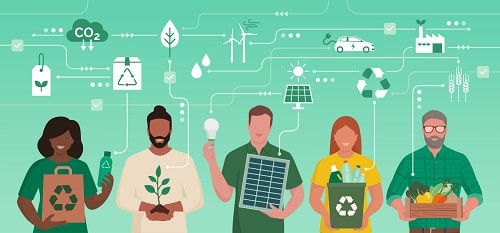
Aligning organisational culture with sustainability: a win, win for the environment and business
By Dr Keith Whitehead, British Safety Council on 04 April 2025
The culture of an organisation is crucial in determining how successfully it implements, integrates and achieves its sustainability and environmental goals and practices. However, there are a number of simple ways of ensuring a positive organisational culture where everyone is fully committed to achieving excellent sustainability performance.